Everyone’s talking AI – What’s up with that?
So, we are hearing a lot about AI these days in the ultrasound world.
Just what is AI, and how does it apply?
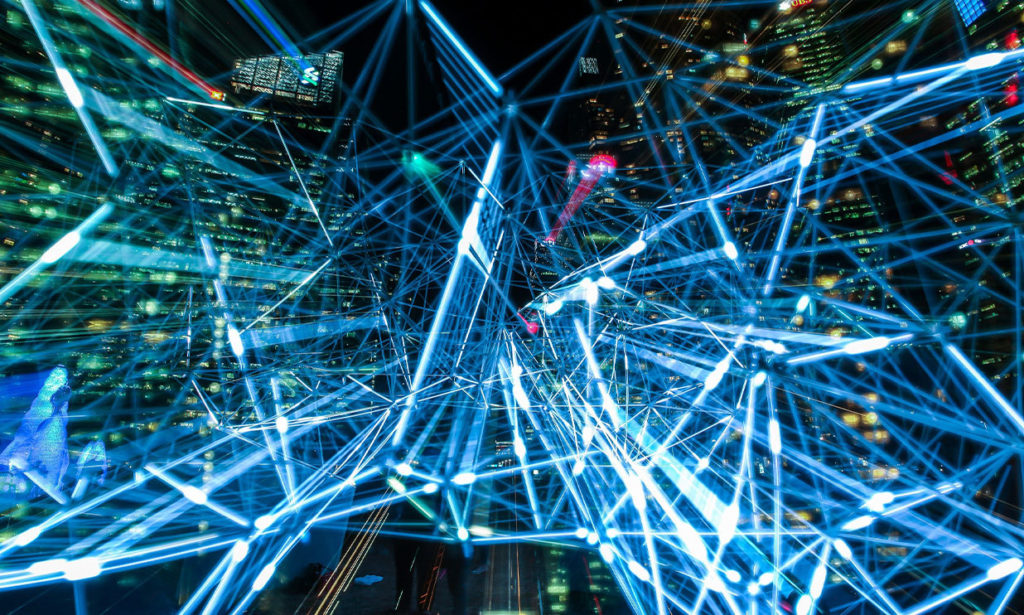
Artificial Intelligence:
noun
Definition of artificial intelligence:
- a branch of computer science dealing with the simulation of intelligent behavior in computers
- the capability of a machine to imitate intelligent human behavior
https://www.merriam-webster.com/dictionary/artificial%20intelligence
There are ultrasound products available today that employ AI to recognize certain aspects of an ultrasound image. Once specific algorithms are trained through thousands of image acquisitions and programmed, the ultrasound system is able use this on-board intelligence to determine the quality of a specific image that the system has been trained to analyze. Also, the system may even perform calculations that, in our past lives, were performed by hand. These products may just outline pathologies like B lines in the lung or, they may determine contours of the left ventricle in both diastole and systole with a mathematical calculation applied to predict an accurate ejection fraction and more…
Cool stuff for sure! Valuable time is saved because the sonographer or physician doesn’t have to trace the left ventricle manually to obtain the information or, for that matter, just guess. The AI tools are specifically becoming more helpful to those with limited experience in learning certain applications in medicine.
Where are these AI applications headed?
First of all, we need a basic understanding of the terms and their exact meanings.
To help us with this, I have asked our Principal Machine Learning Scientist, Allen Lu, to help me understand the differences between Artificial Intelligence (AI), Machine Learning, and Deep Learning. After a brief interview with Allen, some of the confusion I had is now cleared up.
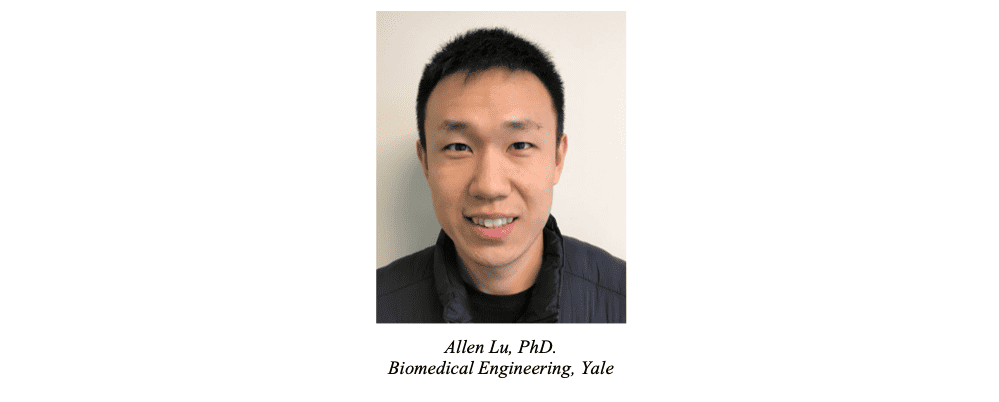
Our interview:
EchoNous: Can you tell me Allen in a few words just what Artificial Intelligence (AI) really means?
Allen: So, Artificial Intelligence or AI, is a broad term that describes systems that are designed to simulate human intelligence or mimic human behavior. These systems can range from self-driving cars to robotic arms on the factory floor.
EchoNous: So how does AI differ from Machine Learning?
Allen: Machine learning is actually a type of AI, where a machine self-learns from observations or data to make a decision or prediction. Typically, in medical imaging, machine learning algorithms learn in a supervised manner, where the model is taught with example images and corresponding expert annotations. However, there are other ways for the model to learn, such as unsupervised learning, semi-supervised learning, or reinforcement learning.
EchoNous: Can you help me better understand then the difference between AI and Deep Learning?
Allen: Deep learning is a specific type of machine learning. Most machine learning algorithms are considered “shallow algorithms” that are only capable of learning simple tasks. However, in 2012, a research group from the University of Toronto developed a deep learning algorithm that vastly outperformed earlier machine learning algorithms. As hinted from the name, deep learning can be thought of as stacking many “shallow” machine learning models together, allowing the overall “deeper” model to learn more complex tasks. This was made possible in 2012 with advancements in deep learning training design and the advent of computational resources such as the graphics processing unit (GPU). Now in 2019, you can find deep learning models automatically processing satellite imagery, generating artwork, understanding text and, of course, interpreting ultrasound images.
EchoNous: Now that you have cleared some of the fog on the subject for me, where do you see all of this headed in the areas of medicine. Specifically, I am interested in ultrasound and the applications applied to making ultrasound more applicable to the less trained individual users.
Allen: This is a great question. I think the number one issue in the application of deep learning for medical or ultrasound imaging is patient safety. The biggest weakness with deep learning is that it is a “black box” or not interpretable, and this presents a problem for erroneous interpretations. Our team here at EchoNous is focused on developing algorithms that are both accurate and understandable for the user. You may have seen articles about robots taking over jobs, but at EchoNous, we aim to partner with our clinicians to ensure patient safety while empowering them with more advanced, powerful diagnostic tools.
Speaking of empowering our clinicians, I see machine learning having a huge role in making ultrasound more widely used in clinical practice, and I see this happening in two ways. First, machine learning will help reduce the steep learning curve for new inexperienced users. For example, this can be done with real-time machine learning-based algorithms that can provide guidance or feedback to the new user. Second, machine learning will help reduce user variability in ultrasound image analysis; this variability can stem from user training, experience, or a lack of image quality. In addition, machine learning-based image analysis provides consistent, objective results that can be reproduced in clinical practice. So overall, I believe reducing the learning curve and establishing objectivity would greatly add to the value of ultrasound in clinical practice.
EchoNous: Wow! Thanks Allen for the clarification. I am particularly interested in the last part of your answer to the final question, which I will go into more detail in this article. Thanks again for taking a few minutes to speak with me.
—
For years I have worked with companies that are interested in bringing ultrasound to new users in everyday medical practice in addition to delivering ultrasound to those outside of the radiology and cardiology world. To a large extent, that has happened, but we can do even more….much more! As a sonographer for approximately 40 years, I have seen so many changes and developments in all areas of medical imaging. Having worked with early CT scanners, X-Ray machines, and the very first digital angiography subtraction devices, I was drawn to the beauty and simplicity of ultrasound. Of course, the lack of ionizing radiation was a major attraction as well.
In the very first of my Backroom Forms, I stated that I believe there are primarily three reasons that prevent clinicians, first responders, and nurse practitioners from employing ultrasound in their everyday practice.
Cost – Training – Time
From the last part of the interview with Allen, I am excited about the possibilities in the Training and the Time aspects.
From my experience attending and teaching many training workshops over the years, it is clear to me that a few days of training without consistent follow-up and practice with ultrasound leads to frustration and a lack of ongoing use of the modality. The most interesting part of my conversation with Allen addresses these concerns:
“Speaking of empowering our clinicians, I see machine learning having a huge role in making ultrasound more widely used in clinical practice, and I see this happening in two ways.
First, machine learning will help reduce the steep learning curve for new inexperienced users. This can be done with real-time machine learning-based algorithms providing guidance or feedback to new users.
Second, machine learning will help reduce user variability in ultrasound image analysis and provide consistent, objective results that can be reproduced in clinical practice”.